I am trying to estimate a random intercept cross lagged panel model (RI-CLPM), as recommended by Hamaker, Kuiper, and Grasman (2015). This model separates each person’s score on a variable at each wave into the group mean for that wave, an individual’s stable score over all waves (the random intercept) and then an individual level deviation at each wave from the score expected by adding the group-wave-mean and individual trait. In graphic form, the RI-CLPM model for a three-wave panel is:
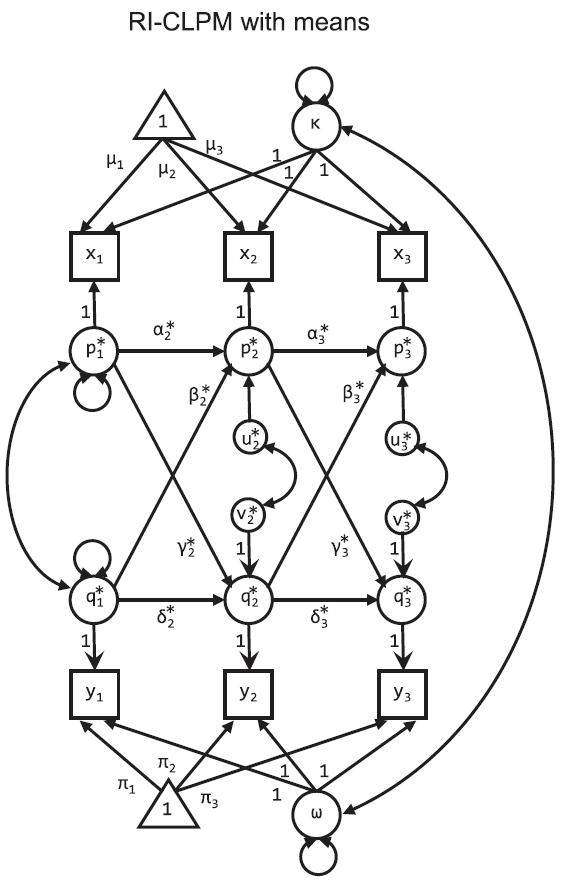
Using the notation of the graph, I estimated the following model (translated from Hamaker et al.'s code for MPlus for RI-CLPMs):
sem (P2 Q2 <- P1 Q1)(P3 Q3 <- P2 Q2)(K -> x1@1 x2@1 x3@1)(W -> y1@1 y2@1 y3@1)(P1 -> x1@1)(P2 -> x2@1)(P3 -> x3@1)(Q1 -> y1@1)(Q2 -> y2@1)(Q3 -> y3@1), cov(K*W P1*Q1 e.P2*e.Q2 e.P3*e.Q3) covstruct(e.P2 e.Q2 e.P3 e.Q3 e.x1 e.x2 e.x3 e.y1 e.y2 e.y3, zero)
Unfortunately, I get the following error message:
model not identified;
too many latent variables
r(503);
Can anyone spot what am I doing wrong here?
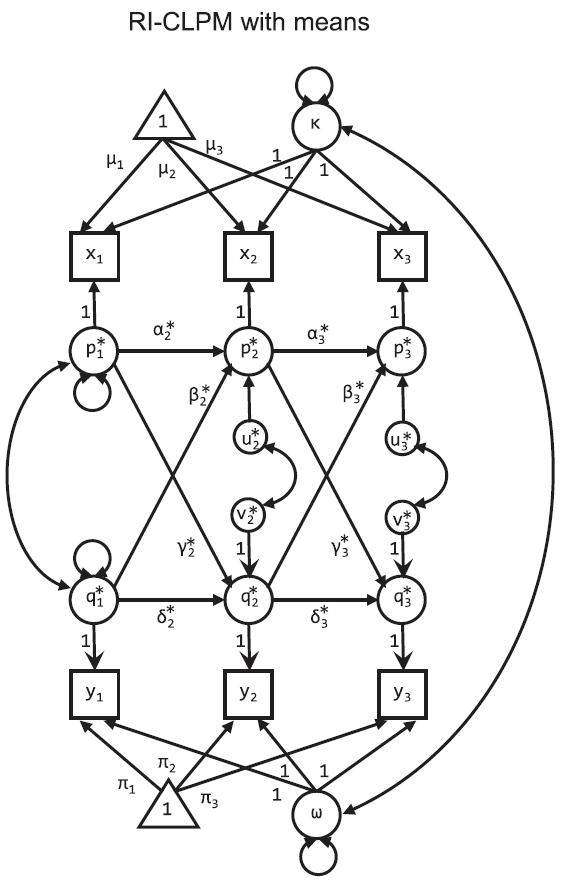
Using the notation of the graph, I estimated the following model (translated from Hamaker et al.'s code for MPlus for RI-CLPMs):
sem (P2 Q2 <- P1 Q1)(P3 Q3 <- P2 Q2)(K -> x1@1 x2@1 x3@1)(W -> y1@1 y2@1 y3@1)(P1 -> x1@1)(P2 -> x2@1)(P3 -> x3@1)(Q1 -> y1@1)(Q2 -> y2@1)(Q3 -> y3@1), cov(K*W P1*Q1 e.P2*e.Q2 e.P3*e.Q3) covstruct(e.P2 e.Q2 e.P3 e.Q3 e.x1 e.x2 e.x3 e.y1 e.y2 e.y3, zero)
Unfortunately, I get the following error message:
model not identified;
too many latent variables
r(503);
Can anyone spot what am I doing wrong here?
Comment